Quantitative Data: Types, Collection Methods, Analysis, and Visualization Techniques
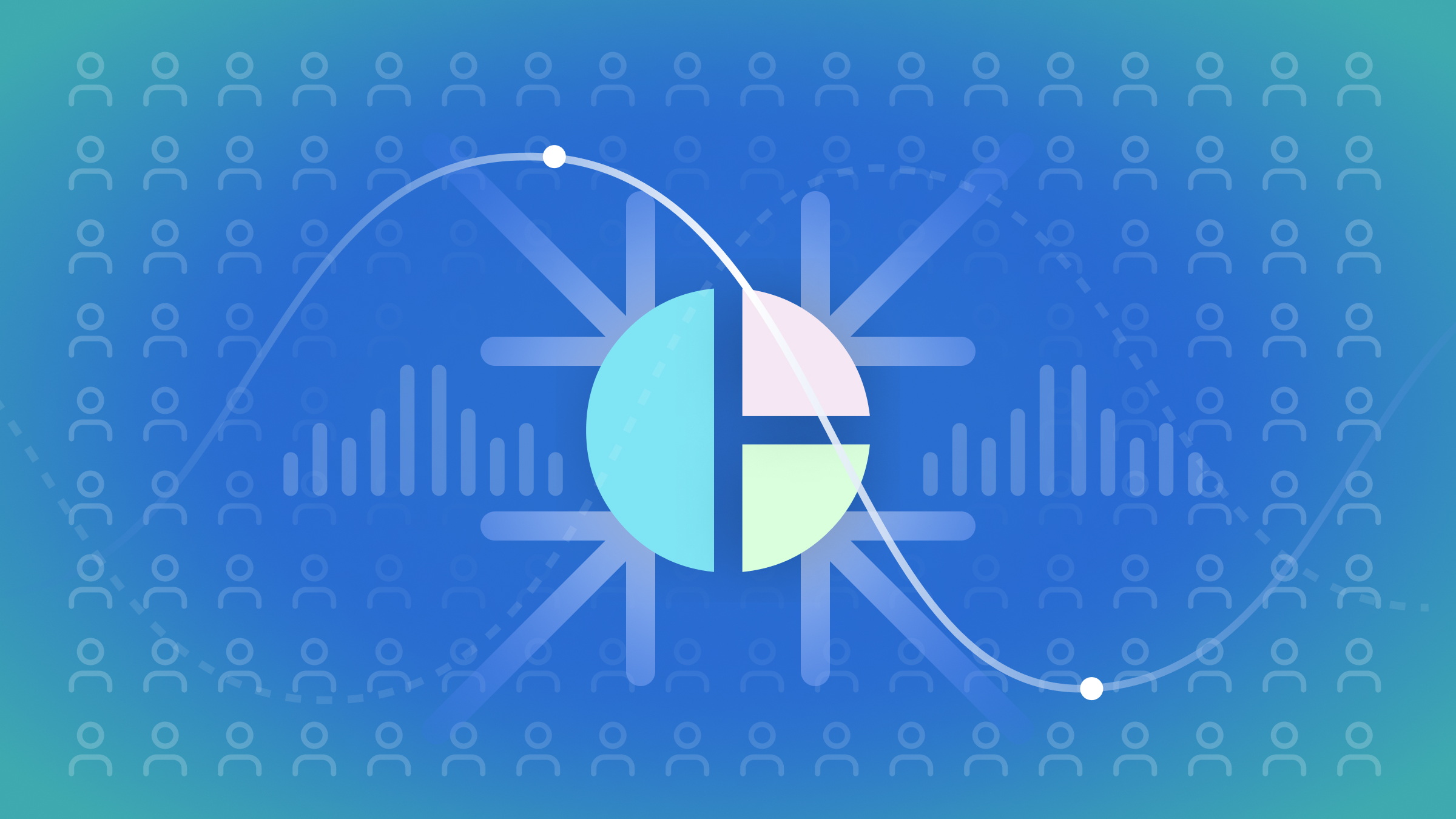
Quantitative Data: Types, Collection Methods, Analysis, and Visualization Techniques
Quantitative data is a crucial component of data-driven decision making in research, business, and many other fields. But what exactly is quantitative data? How is it collected, analyzed, and visualized to extract valuable insights? This article provides a comprehensive overview of quantitative data, including its definition, types, collection methods, analysis techniques, and visualization strategies. Whether you’re a researcher, data analyst, or business professional, understanding quantitative data is essential for making informed, evidence-based decisions.
Key Takeaways
- Quantitative data is numerical, objective, and suitable for statistical analysis
- Discrete data takes fixed values, while continuous data can take any value in a range
- Quantitative data can be collected through surveys, experiments, observational studies, simulations, and existing databases
- Analysis techniques include descriptive statistics, inferential statistics, and data mining
- Effective visualization is crucial for communicating insights from quantitative data
What is Quantitative Data?
Quantitative data is information that can be quantified, counted, or measured, and given a numerical value. It is expressed in numbers rather than natural language description.
Some key characteristics of quantitative data include:
- Numerical nature
- Objective and structured
- Suitable for mathematical and statistical analysis
- Allows for generalization to larger populations
Quantitative vs Qualitative Data
Quantitative data differs from qualitative data in several key ways:
Quantitative Data | Qualitative Data |
---|---|
Numerical | Descriptive |
Objective | Subjective |
Structured | Unstructured |
Statistical analysis | Thematic analysis |
Generalization | In-depth understanding |
While quantitative data focuses on numbers and statistical analysis, qualitative data deals with descriptions, characteristics, and thematic exploration. Both types of data are valuable and often used together in research for a comprehensive understanding of a topic.
Types of Quantitative Data
Quantitative data can be classified into two main types: discrete and continuous.
Discrete Data
Discrete data can only take on certain fixed values, typically whole numbers. Examples include:
- Number of customers
- Number of defective products
- Shoe size
- Number of website visits
Continuous Data
Continuous data can take on any value within a range. It can be further divided into interval and ratio data.
Interval Data
Interval data has equal intervals between values, but no true zero point. Examples include:
- Temperature in Celsius or Fahrenheit
- Dates on a calendar
- Test scores (e.g., IQ, SAT)
Ratio Data
Ratio data has equal intervals and a true zero point, allowing for meaningful ratios. Examples include:
- Height
- Weight
- Time
- Income
Quantitative Data Collection Methods
There are several methods for collecting quantitative data, including:
Surveys and Questionnaires
Surveys and questionnaires are widely used to gather quantitative data from a large sample. They can be administered online, by phone, or in person.
Experiments
Experiments involve manipulating one variable to observe its effect on another variable. They are commonly used in scientific research and can be controlled or randomized.
Observational Studies
Observational studies involve collecting data without manipulating variables. Researchers observe and record data in a natural setting.
Simulations
Simulations involve creating a model of a real-world system and collecting data from the model. They are useful when real-world experimentation is impractical or unethical.
Existing Databases
Researchers can also utilize existing databases, such as government statistics or company records, to obtain quantitative data.
Quantitative Data Analysis Techniques
Once quantitative data is collected, various statistical techniques can be used for analysis, including:
Descriptive Statistics
Descriptive statistics summarize and describe the main features of a dataset, such as central tendency (mean, median, mode) and dispersion (range, variance, standard deviation).
Inferential Statistics
Inferential statistics use sample data to make generalizations about a larger population. Techniques include hypothesis testing, confidence intervals, and regression analysis.
Data Mining
Data mining involves discovering patterns and relationships in large datasets using techniques like clustering, association rules, and decision trees.
Quantitative Data Visualization
Visualizing quantitative data helps communicate insights effectively. Some common visualization techniques include:
Bar Charts and Histograms
Bar charts compare categorical data, while histograms show the distribution of continuous data.
Line Graphs
Line graphs display trends or changes over time.
Scatter Plots
Scatter plots show the relationship between two continuous variables.
Heat Maps
Heat maps use color intensity to represent values in a matrix.
FAQs
Quantitative data is used in various fields, such as: * Business: Sales figures, customer metrics, financial data * Healthcare: Clinical trial results, patient metrics, treatment effectiveness * Education: Test scores, graduation rates, student performance metrics * Social Sciences: Survey results, demographic data, economic indicators
Combining quantitative and qualitative data, known as mixed-methods research, provides a more comprehensive understanding of a topic. Quantitative data offers generalizability and statistical evidence, while qualitative data provides context and in-depth insights.
Some common challenges include: * Data quality issues (e.g., missing data, outliers, measurement errors) * Ensuring data privacy and security * Selecting appropriate analysis techniques * Interpreting results accurately and avoiding misleading conclusions
Conclusion
By understanding the types, collection methods, analysis techniques, and visualization strategies for quantitative data, researchers and professionals can leverage this valuable information source to drive evidence-based decision making and solve complex problems.